AGGREGATIVE CONTINGENT ESTIMATION (ACE) Related Article(s) • Fortune telling: Crowds surpass pundits • Does anyone make accurate geopolitical predictions? • Could you be a ‘super-forecaster’? • Some people really are better at predicting the future. Here are the traits they have in common • Teams Better Than Individuals at Intelligence Analysis, Research Finds • So You Think You're Smarter Than A CIA Agent IMPROVING FORECASTING THROUGH THE WISDOM OF CROWDS Philip Tetlock in The New York Times ...
In 2006, Philip E. Tetlock published a landmark book called “Expert Political Judgment.” While his findings obviously don’t apply to me, Tetlock demonstrated that pundits and experts are terrible at making predictions. But Tetlock is also interested in how people can get better at making forecasts. His subsequent work helped prompt people at one of the government’s most creative agencies, the Intelligence Advanced Research Projects Agency, to hold a forecasting tournament to see if competition could spur better predictions. In the fall of 2011, the agency asked a series of short-term questions about foreign affairs, such as whether certain countries will leave the euro, whether North Korea will re-enter arms talks, or whether Vladimir Putin and Dmitri Medvedev would switch jobs. They hired a consulting firm to run an experimental control group against which the competitors could be benchmarked. Five teams entered the tournament, from places like M.I.T., Michigan and Maryland. Tetlock and his wife, the decision scientist Barbara Mellers, helped form a Penn/Berkeley team, which bested the competition and surpassed the benchmarks by 60 percent in Year 1. How did they make such accurate predictions? In the first place, they identified better forecasters. It turns out you can give people tests that usefully measure how open-minded they are. For example, if you spent $1.10 on a baseball glove and a ball, and the glove cost $1 more than the ball, how much did the ball cost? Most people want to say that the glove cost $1 and the ball 10 cents. But some people doubt their original answer and realize the ball actually costs 5 cents. Tetlock and company gathered 3,000 participants. Some got put into teams with training, some got put into teams without. Some worked alone. Some worked in prediction markets. Some did probabilistic thinking and some did more narrative thinking. The teams with training that engaged in probabilistic thinking performed best. The training involved learning some of the lessons included in Daniel Kahneman’s great work, “Thinking, Fast and Slow.” For example, they were taught to alternate between taking the inside view and the outside view. Suppose you’re asked to predict whether the government of Egypt will fall. You can try to learn everything you can about Egypt. That’s the inside view. Or you can ask about the category. Of all Middle Eastern authoritarian governments, what percentage fall in a given year? That outside view is essential. Most important, participants were taught to turn hunches into probabilities. Then they had online discussions with members of their team adjusting the probabilities, as often as every day. People in the discussions wanted to avoid the embarrassment of being proved wrong. In these discussions, hedgehogs disappeared and foxes prospered. That is, having grand theories about, say, the nature of modern China was not useful. Being able to look at a narrow question from many vantage points and quickly readjust the probabilities was tremendously useful. The Penn/Berkeley team also came up with an algorithm to weigh the best performers. Let’s say the top three forecasters all believe that the chances that Italy will stay in the euro zone are 0.7 (with 1 being a certainty it will and 0 being a certainty it won’t). If those three forecasters arrive at their judgments using different information and analysis, then the algorithm synthesizes their combined judgment into a 0.9. It makes the collective judgment more extreme. This algorithm has been extremely good at predicting results. Tetlock has tried to use his own intuition to beat the algorithm but hasn’t succeeded. In the second year of the tournament, Tetlock and collaborators skimmed off the top 2 percent of forecasters across experimental conditions, identifying 60 top performers and randomly assigning them into five teams of 12 each. These “super forecasters” also delivered a far-above-average performance in Year 2. Apparently, forecasting skill cannot only be taught, it can be replicated. Tetlock is now recruiting for Year 3. (You can match wits against the world by visiting www.goodjudgmentproject.com.) He believes that this kind of process may help depolarize politics. If you take Republicans and Democrats and ask them to make a series of narrow predictions, they’ll have to put aside their grand notions and think clearly about the imminently falsifiable. If I were President Obama or John Kerry, I’d want the Penn/Berkeley predictions on my desk. The intelligence communities may hate it. High-status old vets have nothing to gain and much to lose by having their analysis measured against a bunch of outsiders. But this sort of work could probably help policy makers better anticipate what’s around the corner. It might induce them to think more probabilistically. It might make them better foxes. Previous selected Edge Master Classes ...
PARTICIPANTS: Stewart Brand, Biologist, Long Now Foundation; Whole Earth Discipline; Larry Brilliant, M.D. Epidemiologist, Skoll Urgent Threats Fund; John Brockman, Publisher & Editor, Edge; Max Brockman, Literary Agent, Brockman, Inc.; What's Next: Dispatches on the Future of Science; Jason Calacanis, Internet Entrepreneur, Mahalo; George Dyson, Science Historian; Darwin Among the Machines; Jesse Dylan, Film-Maker, Form.tv, FreeForm.tv; Arie Emanuel, Chairman, William Morris Endeavor Entertainment; Sam Harris, Neuroscientist, UCLA; The End of Faith; W. Daniel Hillis, Computer Scientist, Applied Minds; Pattern On The Stone; Thomas Kalil, Deputy Director for Policy for the White House Office of Science and Technology Policy and Senior Advisor for Science, Technology and Innovation for the National Economic Council; Salar Kamangar, Vice President, Product Management, Google; Lawrence Krauss, Physicist, Origins Initiative, ASU; Hiding In The Mirror; John Markoff, Journalist,The New York Times; What The Dormouse Said; Katinka Matson, Cofounder, Edge; Artist, katinkamatson.com; Elon Musk, Physicist, SpaceX, Tesla Motors; Nathan Myhrvold, Physicist, CEO, Intellectual Ventures, LLC, The Road Ahead; Tim O'Reilly, Founder, O'Reilly Media, O'Reilly Radar; Larry Page, CoFounder, Google; Lucy Page Southworth, Biomedical Informatics Researcher, Stanford; Sean Parker,The Founders Fund; CoFounder Napster & Facebook; Ryan Phelan, Founder, DNA Direct; Nick Pritzker, Chairman, Hyatt Development Corporation; Ed Regis, Writer; What Is Life?; Terrence Sejnowski, Computational Neurobiologist, Salk; The Computational Brain; Maria Spiropulu,Physicist, Cern & Caltech; Victoria Stodden, Computational Legal Scholar, Yale Law School; Richard Thaler, Behavioral Economist, U. Chicago; Nudge; Craig Venter,Genomics Researcher; CEO, Synthetic Genomics,A Life Decoded; Nathan Wolfe, Biologist, Global Virus Forecasting Initiative.
PARTICIPANTS: Jeff Bezos, Founder, Amazon.com; John Brockman, Edge Foundation, Inc.; Max Brockman, Brockman, Inc.; George Dyson, Science Historian; Author, Darwin Among the Machines; W. Daniel Hillis, Computer Scientist; Cofounder, Applied Minds; Author, The Pattern on the Stone; Daniel Kahneman, Psychologist; Nobel Laureate, Princeton University; Salar Kamangar, Google; France LeClerc, Marketing Professor; Katinka Matson, Edge Foundation, Inc.; Sendhil Mullainathan, Professor of Economics, Harvard University; Executive Director, Ideas 42, Institute of Quantitative Social Science; Elon Musk, Physicist; Founder, Tesla Motors, SpaceX; Nathan Myhrvold, Physicist; Founder, Intellectual Venture, LLC; Event Photographer; Sean Parker, The Founders Fund; Cofounder: Napster, Plaxo, Facebook; Paul Romer, Economist, Stanford; Richard Thaler, Behavioral Economist, Director of the Center for Decision Research, University of Chicago Graduate School of Business; coauthor of Nudge; Anne Treisman, Psychologist, Princeton University; Evan Williams, Founder, Blogger, Twitter.
PARTICIPANTS: Jeff Bezos, Founder, Amazon.com; Stewart Brand, Cofounder, Long Now Foundation, Author, How Buildings Learn; Sergey Brin, Founder, Google; John Brockman, Edge Foundation, Inc.; Max Brockman, Brockman, Inc.; Peter Diamandis, Space Entrepreneur, Founder, X Prize Foundation; George Dyson, Science Historian; Author, Darwin Among the Machines; W. Daniel Hillis, Computer Scientist; Cofounder, Applied Minds; Author, The Pattern on the Stone; Daniel Kahneman, Psychologist; Nobel Laureate, Princeton University; Dean Kamen, Inventor, Deka Research; Salar Kamangar, Google; Seth Lloyd, Quantum Physicist, MIT, Author,Programming The Universe; Katinka Matson, Cofounder, Edge Foundation, Inc.;Nathan Myhrvold, Physicist; Founder, Intellectual Venture, LLC; Event Photographer;Tim O'Reilly, Founder, O'Reilly Media; Larry Page, Founder, Google; George Smoot, Physicist, Nobel Laureate, Berkeley, Coauthor, Wrinkles In Time; Anne Treisman, Psychologist, Princeton University; Jimmy Wales, Founder, Chair, Wikimedia Foundation (Wikipedia). Philip Tetlock on Edge ...
The question becomes, is it possible to set up a system for learning from history that's not simply programmed to avoid the most recent mistake in a very simple, mechanistic fashion? Is it possible to set up a system for learning from history that actually learns in our sophisticated way that manages to bring down both false positive and false negatives to some degree? That's a big question mark. Nobody has really systematically addressed that question until IARPA, the Intelligence Advanced Research Projects Agency, sponsored this particular project, which is very, very ambitious in scale. It's an attempt to address the question of whether you can push political forecasting closer to what philosophers might call an optimal forecasting frontier. That an optimal forecasting frontier is a frontier along which you just can't get any better.
Introduction Philip Tetlock’s 2005 book Expert Political Judgment: How Good Is It? How Can We Know? demonstrated that accurate long-term political forecasting is, to a good approximation, impossible. The work was a landmark in social science, and its importance was quickly recognized and rewarded in two academic disciplines—political science and psychology. Perhaps more significantly, the work was recognized in the intelligence community, which accepted the challenge of investing significant resources in a search for improved accuracy. The work is ongoing, important discoveries are being made, and Tetlock gives us a chance to peek at what is happening. Tetlock’s current message is far more positive than his earlier dismantling of long-term political forecasting. He focuses on the near term, where accurate prediction is possible to some degree, and he takes on the task of making political predictions as accurate as they can be. He has successes to report. As he points out in his comments, these successes will be destabilizing to many institutions, in ways both multiple and profound. With some confidence, we can predict that another landmark of applied social science will soon be reached. Daniel Kahneman, recipient of the Nobel Prize in Economics, 2002, is the Eugene Higgins Professor of Psychology Emeritus at Princeton University and author of Thinking Fast and Slow.
HOW TO WIN AT FORECASTING There's a question that I've been asking myself for nearly three decades now and trying to get a research handle on, and that is why is the quality of public debate so low and why is it that the quality often seems to deteriorate the more important the stakes get? About 30 years ago I started my work on expert political judgment. It was the height of the Cold War. There was a ferocious debate about how to deal with the Soviet Union. There was a liberal view; there was a conservative view. Each position led to certain predictions about how the Soviets would be likely to react to various policy initiatives. One thing that became very clear, especially after Gorbachev came to power and confounded the predictions of both liberals and conservatives, was that even though nobody predicted the direction that Gorbachev was taking the Soviet Union, virtually everybody after the fact had a compelling explanation for it. We seemed to be working in what one psychologist called an "outcome irrelevant learning situation." People drew whatever lessons they wanted from history.
There is quite a bit of skepticism about political punditry, but there's also a huge appetite for it. I was struck 30 years ago and I'm struck now by how little interest there is in holding political pundits who wield great influence accountable for predictions they make on important matters of public policy. The presidential election of 2012, of course, brought about the Nate Silver controversy and a lot of people, mostly Democrats, took great satisfaction out of Silver being more accurate than leading Republican pundits. It's undeniably true that he was more accurate. He was using more rigorous techniques in analyzing and aggregating data than his competitors and debunkers were. But it’s not something uniquely closed-minded about conservatives that caused them to dislike Silver. When you go back to presidential elections that Republicans won, it's easy to find commentaries in which liberals disputed the polls and complained the polls were biased. That was true even in a blow-out political election like 1972, the McGovern-Nixon election. There were some liberals who had convinced themselves that the polls were profoundly inaccurate. It's easy for partisans to believe what they want to believe and political pundits are often more in the business of bolstering the prejudices of their audience than they are in trying to generate accurate predictions of the future. Thirty years ago we started running some very simple forecasting tournaments and they gradually expanded. We were interested in answering a very simple question, and that is what, if anything, distinguishes political analysts who are more accurate from those who are less accurate on various categories of issues? We looked hard for correlates of accuracy. We were also interested in the prior question of whether political analysts can do appreciably better than chance. We found two things. One, it's very hard for political analysts to do appreciably better than chance when you move beyond about one year. Second, political analysts think they know a lot more about the future than they actually do. When they say they're 80 or 90 percent confident they're often right only 60 or 70 percent of the time. There was systematic overconfidence. Moreover, political analysts were disinclined to change their minds when they get it wrong. When they made strong predictions that something was going to happen and it didn’t, they were inclined to argue something along the lines of, "Well, I predicted that the Soviet Union would continue and it would have if the coup plotters against Gorbachev had been more organized," or "I predicted the Canada would disintegrate or Nigeria would disintegrate and it's still, but it's just a matter of time before it disappears," or "I predicted that the Dow would be down 36,000 by the year 2000 and it's going to get there eventually, but it will just take a bit longer." So, we found three basic things: many pundits were hardpressed to do better than chance, were overconfident, and were reluctant to change their minds in response to new evidence. That combination doesn't exactly make for a flattering portrait of the punditocracy. We did a book in 2005 and it's been quite widely discussed. Perhaps the most important consequence of publishing the book is that it encouraged some people within the US intelligence community to start thinking seriously about the challenge of creating accuracy metrics and for monitoring how accurate analysts are–which has led to the major project that we're involved in now, sponsored by the Intelligence Advanced Research Projects Activities (IARPA). It extends from 2011 to 2015, and involves thousands of forecasters making predictions on hundreds of questions over time and tracking in accuracy. Exercises like this are really important for a democracy. The Nate Silver episode illustrates in a small way what I hope will happen over and over again over the next several decades, which is, there are ways of benchmarking the accuracy of pundits. If pundits feel that their accuracy is benchmarked they will be more careful about what they say, they'll be more thoughtful about what they say, and it will elevate the quality of public debate. One of the reactions to my work on expert political judgment was that it was politically naïve; I was assuming that political analysts were in the business of making accurate predictions, whereas they're really in a different line of business altogether. They're in the business of flattering the prejudices of their base audience and they're in the business of entertaining their base audience and accuracy is a side constraint. They don't want to be caught in making an overt mistake so they generally are pretty skillful in avoiding being caught by using vague verbiage to disguise their predictions. They don't say there's a .7 likelihood of a terrorist attack within this span of time. They don't say there's a 1.0 likelihood of recession by the third quarter of 2013. They don't make predictions like that. What they say is if we go ahead with the administration's proposed tax increase there could be a devastating recession in the next six months. "There could be." The word "could" is notoriously ambiguous. When you ask research subjects what "could" means it depends enormously on the context. "We could be struck by an asteroid in the next 25 seconds," which people might interpret as something like a .0000001 probability, or "this really could happen," which people might interpret as a .6 or .7 probability. It depends a lot on the context. Pundits have been able to insulate themselves from accountability for accuracy by relying on vague verbiage. They can often be wrong, but never in error. ~~ There is an interesting case study to be done on the reactions of the punditocracy to Silver. Those who are most upfront in debunking him, holding him in contempt, ridiculing him, and offering contradictory predictions were put in a genuinely awkward situation because they were so flatly disconfirmed. They had violated one of the core rules of their own craft, which is to insulate themselves in vague verbiage–to say, "Well, it's possible that Obama would win." They should have cushioned themselves in various ways with rhetoric. How do people react when they're actually confronted with error? You get a huge range of reactions. Some people just don't have any problem saying, "I was wrong. I need to rethink this or that assumption." Generally, people don't like to rethink really basic assumptions. They prefer to say, "Well, I was wrong about how good Romney's get out to vote effort was." They prefer to tinker with the margins of their belief system (e.g., "I fundamentally misread US domestic politics, my core area of expertise"). A surprising fraction of people are reluctant to acknowledge there was anything wrong with what they were saying. One argument you sometimes hear, and we heard this in the abovementioned episode, but I also heard versions of it after the Cold War. "I was wrong, but I made the right mistake." Dick Morris, the Republican pollster and analyst conceded that he was wrong, but it was the right mistake to make because he was acting, essentially, as a cheerleader for a particular side and it would have been far worse to have underestimated Romney than to have overestimated him. If you have a theory how world politics works that can lead you to value avoiding one error more than the complementary error. You might say, "Well, it was really important to bail out this country because if we hadn't it would have led to financial contagion. There was a risk of losing our money in the bailout, but the risk was offset because I thought the risk of contagion was substantial." If you have a contagion theory of finance that theory will justify putting bailout money at risk. If you have a theory that the enemy is only going to grow bolder if you don't act really strongly against it then you're going to say, "Well, the worst mistake would have been to appease them so we hit them really hard. And even though that led to an expansion of the conflict it would have been far worse if we'd gone down the other path." It's very, very hard to pin them down and that's why these types of level playing field forecasting tournaments can play a vital role in improving the quality of public debate. There are various interesting scientific objections that have been raised to these level playing field forecasting exercises. One line of objection would be grounded more in Nassim Taleb's school of thought, the Black Swan view of history: Where we are in history today is the product of forces that not only no one foresaw, but no one could have foreseen. The epoch transforming events like World War One, nuclear bombs and nuclear missiles to deliver them, and the invention of the Internet–the geopolitical and technological transformational events in history no one foresaw, no one could have foreseen. In this view, history is best understood in tems of a punctuated equilibrium model. There are periods of calm and predictability punctuated by violent exogenous shocks that transform things–sometimes for the better, sometimes for the worse–and these discontinuities are radically unpredictable. What are we doing? Well, in this view, we may be lulling people into a kind of false complacency by giving them the idea that you can improve your foresight to an ascertainable degree within ascertainable time parameters and types of tasks. That's going to induce a false complacency and will cause us to be blindsided all the more violently by the next black swan because we think we have a good probabilistic handle on an erratically unpredictable world–which is an interesting objection and something we have to be on the lookout for. There is, of course, no evidence to support that claim. I would argue that making people more appropriately humble about their ability to predict a short term future is probably, on balance, going to make them more appropriately humble about their ability to predict the long term future, but that certainly is a line of argument that's been raised about the tournament. Another interesting variant of that argument is that it's possible to learn in certain types of tasks, but not in other types of tasks. It's possible to learn, for example, how to be a better poker player. Nate Silver could learn to be a really good poker player. Hedge fund managers tend to be really good poker players, probably because it's good preparation for their job. Well, what does it mean to be a good poker player? You learn to be a good poker player because you get repeated clear feedback and you have a well-defined sampling universe from which the cards are being drawn. You can actually learn to make reasonable probability estimates about the likelihood of various types of hands materializing in poker. Is world politics like a poker game? This is what, in a sense, we are exploring in the IARPA forecasting tournament. You can make a good case that history is different and it poses unique challenges. This is an empirical question of whether people can learn to become better at these types of tasks. We now have a significant amount of evidence on this, and the evidence is that people can learn to become better. It's a slow process. It requires a lot of hard work, but some of our forecasters have really risen to the challenge in a remarkable way and are generating forecasts that are far more accurate than I would have ever supposed possible from past research in this area. Silver's situation is more like poker than geopolitics. He has access to polls that are being drawn from representative samples. The polls have well defined statistical properties. There's a well-defined sampling universe, so he is closer to the poker domain when he is predicting electoral outcomes in advanced democracies with well established polling procedures, well-established sampling methodologies, relatively uncorrupted polling processes. That's more like poker and less like trying to predict the outcome of a civil war in Sub-Saharan Africa or trying to predict H5N1 is going to spread in a certain way or many of the types of events that loom large in geopolitical or technological forecasting. There has long been disagreement among social scientists about how scientific social science can be, and the skeptics have argued that social phenomena are more cloudlike. They don't have Newtonian clocklike regularity. That cloud versus clock distinction has loomed large in those kinds of debates. If world politics were truly clocklike and deterministic then it should in principle be possible for an observer who is armed with the correct theory and correct knowledge of the antecedent conditions to predict with extremely high accuracy what's going to happen next. If world politics is more cloudlike–little wisps of clouds blowing around in the air in quasi random ways–no matter how theoretically prepared the observer is, the observer is not going to be able to predict very well. Let's say the clocklike view posits that the optimal forecasting frontier is very close to 1.0, an R squared very close to 1.0. By contrast, the cloudlike view would posit that the optimal forecasting frontier is not going to be appreciably greater than chance or you're not going to be able to do much better than a dart-throwing chimpanzee. One of the things that we discovered in the earlier work was that forecasters who suspected that politics was more cloudlike were actually more accurate in predicting longer-term futures than forecasters who believed that it was more clocklike. Forecasters who were more modest about what could be accomplished predictably were actually generating more accurate predictions than forecasters who were more confident about what could be achieved. We called these theoretically confident forecasters "hedgehogs." We called these more modest, self-critical forecasters "foxes," drawing on Isaiah Berlin's famous essay, "The Hedgehog and the Fox." Let me say something about how dangerous it is to draw strong inferences about accuracy from isolated episodes. Imagine, for example, that Silver had been wrong and that Romney had become President. And let's say his prediction had been a 0.8 probability two weeks prior to the election that made Romney President. You can imagine what would have happened to his credibility. It would have cratered. People would have concluded that, yes, his Republican detractors were right, that he was essentially an Obama hack, and he wasn't a real scientist. That's, of course, nonsense. When you say there's a .8 probability there's 20 percent chance that something else could happen. And it should reduce your confidence somewhat in him, but you shouldn't abandon him totally. There's a disciplined Bayesian belief adjustment process that's appropriate in response to mis-calibrated forecasts. What we see instead is overreactions. Silver would either be a fool if he'd gotten it wrong or he's a god if he gets it right. He's neither a fool nor a god. He's a thoughtful data analyst who knows how to work carefully through lots of detailed data and aggregate them in sophisticated ways and get a bit of a predictive edge over many, but not all of his competitors. Because there are other aggregators out there who are doing as well or maybe even a little bit better, but their methodologies are quite strikingly similar and they're relying on a variant of the wisdom of the crowd, which is aggregation. They're pooling a lot of diverse bits of information and they're trying to give more weight to those bits of information that have a good historical track record of having been accurate. It's a weighted averaging kind of process essentially and that's a good strategy. I don't have a dog in this theoretical fight. There's one school of thought that puts a lot of emphasis on the advantages of blink, on the advantages of going with your gut. There's another school of thought that puts a lot of emphasis on the value of system two overrides, self-critical cognition–giving things over a second thought. For me it is really a straightforward empirical question of what are the conditions under which each style of thinking works better or worse? In our work on expert political judgment we have generally had a hard time finding support for the usefulness of fast and frugal simple heuristics. It's generally the case that forecasters who are more thoughtful and self-critical do a better job of attaching accurate probability estimates to possible futures. I'm sure there are situations when going with a blink may well be a good idea and I'm sure there are situations when we don't have time to think. When you think there might be a tiger in the jungle you might want to move very fast before you fully process the information. That's all well known and discussed elsewhere. For us, we're finding more evidence for the value of thoughtful system two overrides, to use Danny Kahneman's terminology. Let's go back to this fundamental question of what are we capable of learning from history and are we capable of learning anything from history that we weren't already ideologically predisposed to learn? As I mentioned before, history is not a good teacher and we see what a capricious teacher history is in the reactions to Nate Silver in the 2012 election forecasting. He's either a genius or he's an idiot and we need to have much more nuanced, well-calibrated reactions to episodes of this sort. ~~ The intelligence community is responsible, of course, for providing the US Government with timely advice about events around the world and they frequently get politically clobbered virtually whenever they make mistakes. There are two types of mistakes you can make, essentially. You can make a false positive prediction or you can make a false negative prediction. What would a false positive prediction look like? Well, the most famous recent false positive prediction is probably the false positive on weapons of mass destruction in Iraq, which led to a trillion-plus dollar war. What about famous false negative predictions? Well, a lot of people would call 9/11 a serious false negative. The intelligence community oscillates back and forth in response to these sharp political critiques that are informed by hindsight and one of the things that we know from elementary behaviorism as well as from work in organizational learning is that rats, people and organizations do respond to rewards and punishments. If an organization has been recently clobbered for making a false positive prediction, that organization is going to make major efforts to make sure it doesn't make another false positive. They're going to be so sure that they might make a lot more false negatives in order to avoid that. "We're going to make sure we're not going to make a false positive even if that means we're going to underestimate the Iranian nuclear program." Or "We're going to be really sure we don't make a false negative even if that means we have false alarms of terrorism for the next 25 years." The question becomes, is it possible to set up a system for learning from history that's not simply programmed to avoid the most recent mistake in a very simple, mechanistic fashion? Is it possible to set up a system for learning from history that actually learns in our sophisticated way that manages to bring down both false positive and false negatives to some degree? That's a big question mark. Nobody has really systematically addressed that question until IARPA, the Intelligence Advanced Research Projects Activities, sponsored this particular project, which is very, very ambitious in scale. It's an attempt to address the question of whether you can push political forecasting closer to what philosophers might call an optimal forecasting frontier. That an optimal forecasting frontier is a frontier along which you just can't get any better. You can't get false positives down anymore without having more false negatives. You can't get false negatives down anymore without having more false positives. That's just the optimal state of prediction unless you subscribe to an extremely clocklike view of the political economic, technological universe. If you subscribe to that you might believe the optimal forecasting frontier is 1.0 and that godlike omniscience is possible. You never have to tolerate any false positives or false negatives. There are very few people on the planet, I suspect, who believe that to be true of our world. But you don't have to go all the way to the cloudlike extreme and say that we are all just radically unpredictable. Most of us are somewhere in between clocklike and cloudlike, but we don't know for sure where we are in that distribution and IARPA is helping us to figure out where we are. It's fascinating to me that there is a steady public appetite for books that highlight the feasibility of prediction like Nate Silver and there's a deep public appetite for books like Nassim Taleb's The Black Swan, which highlight the apparent unpredictability of our universe. The truth is somewhere in between and IARPA style tournaments are a method of figuring out roughly where we are in that conceptual space at the moment, with the caveat that things can always change suddenly. I recall Daniel Kahneman having said on a number of occasions that when he's talking to people in large organizations, private or public sector, he challenges the seriousness of their commitment to improving judgment and choice. The challenge takes the following form–would you be willing to devote one percent of your annual budget to efforts to improve judgment and choice? And to the best of my knowledge, I don't think he's had any takers yet. ~~ One of the things I've discovered in my work on assessing the accuracy of probability judgment is that there is much more eagerness in participating in these exercises among people who are younger and lower in status in organizations than there is among people who are older and higher in status in organizations. This doesn't require great psychological insight to understand this. You have a lot more to lose if you're senior and well established and your judgment is revealed to be far less well calibrated than those of people who are far junior to you. Level playing field forecasting exercises are radically meritocratic. They put everybody on the same playing field. Tom Friedman no longer has an advantage over an unknown columnist, or that matter, an unknown graduate student. If Tom Friedman's subjective probability estimate for how things are going in the Middle East are less accurate than those of the graduate student at Berkeley, the forecasting tournament just cranks through the numbers and that's what you discover. These are potentially radically status-destabilizing interventions. They have the potential to destabilize status relationships within government agencies. They have the potential to destabilize the status within the private sector. The primary claim that people higher in status organizations have to holding their positions is cognitive in nature. They know better. They know things that the people below them don't know. And in so far as forecasting exercises are probative and give us insight into who knows what about what, they are, again, status destabilizing. From a sociological point of view, it's a minor miracle that this forecasting tournament is even occurring. Government agencies are not supposed to sponsor exercises that have the potential to embarrass them. It would be embarrassing if it turns out that thousands of amateurs working on relatively small budgets are able to outperform professionals within a multibillion-dollar bureaucracy. That would be destabilizing. If it turns out that junior analysts within that multibillion-dollar bureaucracy can perform better than people high up in the bureaucracy that would be destabilizing. If it turns out that the CEO is not nearly as good as people two or three tiers down in perceiving strategic threats to the business, that's destabilizing. Things that bring transparency to judgment are dangerous to your status. You can make a case for this happening in medicine, for example. In so far as evidence based medicine protocols become increasingly influential, doctors are going to rely more and more on the algorithms–otherwise they're not going to get their bills paid. If they're not following the algorithms, it's not going to be reimbursable. When the healthcare system started to approach 20 to 25 percent of the GDP, very powerful economic actors started pushing back and demanding accountability for medical judgment. ~~ The long and the short of the story is that it's very hard for professionals and executives to maintain their status if they can't maintain a certain mystique about their judgment. If they lose that mystique about their judgment, that's profoundly threatening. My inner sociologist says to me that when a good idea comes up against entrenched interests, the good idea typically fails. But this is going to be a hard thing to suppress. Level playing field forecasting tournaments are going to spread. They're going to proliferate. They're fun. They're informative. They're useful in both the private and public sector. There's going to be a movement in that direction. How it all sorts out is interesting. To what extent is it going to destabilize the existing pundit hierarchy? To what extent is it going to destabilize who the big shots are within organizations? The Intelligence Advance Research Projects Agency about two years ago committed to supporting five university based research teams and funded their efforts to recruit forecasters, set up websites for eliciting forecasts, hire statisticians for aggregating forecasts, and conduct a variety of experiments on factors that might either make forecasters more accurate or less accurate. For about a year and half we've been doing actual forecasting. There are two aspects of this. There's a horserace aspect to it and there's a basic science aspect. The horserace aspect is which team is more accurate? Which team is generating probability judgments that are closer to reality? What does it mean to generate a probability judgment closer to reality? If I say there is a .9 likelihood of Obama winning reelection and Nate Silver says there's a .8 likelihood of Obama reelection and Obama wins reelection, the person who said .9 is closer than the person who said .8. So that person deserves a better accuracy score. If someone said .2, they get a really bad accuracy score. There are some statistical procedures that we use for a method of scoring probability judgment. It's called Brier scoring. Brier scoring is what we are using right now for assessing accuracy, but there are many other statistical techniques that can be applied. Our conclusions are robust across them. But the idea is to get people to make explicit probability judgments, score them against reality. Yet, to make this work you also have to pose questions that could be resolved in a clear-cut way. You can't say, "I think there could be a lot of instability in Afghanistan after NATO forces withdraw in 2014." That's not a good question. The questions need to pass what some psychologists have called the "Clairvoyance Test." A good question would be one that I could take and I could hand it to a genuine clairvoyant and I could ask that clairvoyant, "What happened? What's the true answer?" A clairvoyant could look into the future and tell me about it. The clairvoyant wouldn't have to come back to me and say, "What exactly do you mean by 'could'?" or "What exactly do you mean by 'increased violence' or 'increased instability' or 'unrest'?" or whatever the other vague phrases are. We would have to translate them into something testable. ~~ Things that bring transparency to judgment are dangerous to your status. You can make a case for this happening in medicine, for example. In so far as evidence based medicine protocols become increasingly influential, doctors are going to rely more and more on the algorithms–otherwise they're not going to get their bills paid. If they're not following the algorithms, it's not going to be reimbursable. When the healthcare system started to approach 20 to 25 percent of the GDP, very powerful economic actors started pushing back and demanding accountability for medical judgment.An important part of this forecasting tournament is moving from interesting issues to testable propositions and this is an area where we discover very quickly where people don't think the way that Karl Popper thought they should think–like falsificationists. We don't naturally look for evidence that could falsify our hunches, and passing the Clairvoyance Test requires doing that. If you think that the Eurozone is going to collapse–if you think it was a really bad idea to put into common currency economies at very different levels of competitiveness, like Greece and Germany (that was a fundamentally unsound macroeconomic thing to do and the Eurozone is doomed), that's a nice example of an emphatic but untestable hedgehog kind of statement. It may be true, but it's not very useful for our forecasting tournament. To make a forecasting tournament work we have to translate that hedgehog like hunch into a testable proposition like will Greece leave the Eurozone or formally withdraw from the Eurozone by May 2013? Or will Portugal? You need to translate the abstract interesting issue into testable propositions and then you need to get lots of thoughtful people to make probability judgments in response to those testable proposition questions. You need to do that over, and over, and over again. Hedgehogs are more likely to embrace fast and frugal heuristics that are in the spirit of "Blink." If you have a hedgehog like framework, you're more likely to think that people who have mastered that framework should be able to diagnose situations quite quickly and reach conclusions quite confidently. Those things tend to co-vary with each other. For example, if you have a generic theory of world politics known as "realism" and you believe when there's a dominant power being threatened by a rising power–say the United States being threatened by China–it's inevitable that those two countries will come to blows in some fashion. If you believe that, then Blink will come more naturally to you as a forecasting strategy. If you're a fox and you believe there's some truth to the generalization that rising powers and hegemons tend to come into conflict with each other, but there are lots of other factors in play in the current geopolitical environment that make it less likely that China and the United States will come into conflict. That doesn't allow blink anymore, does it? It leads to "on the one hand, and on the other" patterns of reasoning–and you've got to strike some kind of integrative resolution of the conflicting arguments. In the IARPA tournament, we're looking at a number of strategies for improving prediction. Some of them are focused on the individual psychological level of analysis. Can we train people in certain principles of probabilistic reasoning that will allow them to become more accurate? The answer is, to some degree we can. Can we put them together in collaborative teams that will bring out more careful self-critical analysis? To some degree we can. Those are interventions at the individual level of analysis. Then the question is, you've got a lot of interesting predictions at the individual level, what are you going to do with them? How are you going to combine them to make a formal prediction in the forecasting tournament? It's probably a bad idea to take your best forecaster and submit that person's forecasts. You probably want something a little more statistically stable in that. That carries us over into the wisdom of the crowd argument. The famous Francis Galton country fair episode in which the average of 500 or 600 fair goers making a prediction about the weight of an ox. I forget the exact numbers, but let's say the estimated the average prediction was 1,100. The individual predictions were anywhere from 300 to 14,000. When we trim outliers and average, it came to 1,103 and the true answer was 1,102. The average was more accurate than all of the individuals from whom the average was derived. I haven't got all the details right there, but that's a stylized representation of the aggregation argument. There is some truth to that in the IARPA tournament. That simple averaging of the individual forecasters helps. But you can take it further, you can go beyond individual averaging and you can move to more complex weighted averaging kinds of formulas of the sort, for example, that Nate Silver and various other polimetricians were using in the 2012 election. But we're not aggregating polls anymore; we're aggregating individual forecasters in sneaky and mysterious ways. Computers are an important part of this story. In "Moneyball" algorithms destabilized the status hierarchy. You remember in the movie, there was this nerdy kid amidst the seasoned older baseball scouts and the nerdy kid was more accurate than the seasoned baseball scouts. It created a lot of friction there. This is a recurring theme in the psychological literature–the tension between human based forecasting and machine or algorithm based forecasting. It goes back to 1954. Paul Meehl wrote on clinical versus actuarial prediction in which clinical psychologists and psychiatrists' predictions were being compared to various algorithms. Over the last 58 years there have been hundreds of studies done comparing human based prediction to algorithm or machine based prediction and the track record doesn't look good for people. People just keep getting their butts kicked over and over again. We don't have geopolitical algorithms that we're comparing our forecasters to, but we're turning our forecasters into algorithms and those algorithms are outperforming the individual forecasters by substantial margins. There's another thing you can do though and it's more the wave of the future. And that is, you can go beyond human versus machine or human versus algorithm comparison or Kasparov versus Deep Blue (the famous chess competition) and ask, how well could Kasparov play chess if Deep Blue were advising him? What would the quality of chess be there? Would Kasparov and Deep Blue have an FIDE chess rating of 3,500 as opposed to Kasparov's rating of, say, 2,800 and the machines rating of, say, 2,900? That is a new and interesting frontier for work and it's one we're experimenting with. In our tournament, we've skimmed off the very best forecasters in the first year, the top two percent. We call them "super forecasters." They're working together in five teams of 12 each and they're doing very impressive work. We're experimentally manipulating their access to the algorithms as well. They get to see what the algorithms look like, as well as their own predictions. The question is–do they do better when they know what the algorithms are or do they do worse? There are different schools of thought in psychology about this and I have some very respected colleagues who disagree with me on it. My initial hunch was that they might be able to do better. Some very respected colleagues believe that they're probably going to do worse. The most amazing thing about this tournament is that it exists because it is so potentially status destabilizing. Another amazing and wonderful thing about this tournament is how many really smart, thoughtful people are willing to volunteer, essentially enormous amounts of time to make this successful. We offer them a token honorarium. We're paying them right now $150 or $250 a year for their participation. The ones who are really taking it seriously–it's way less the minimum wage. And they're some very thoughtful professionals who are participating in this. Some political scientists I know have had some disparaging things to say about the people who might participate in something like this and one phrase that comes to mind is "unemployed news junkies." I don't think that's a fair characterization of our forecasters. Certainly the most actively engaged of our forecasters are really pretty awesome. They're very skillful at finding information, synthesizing it, and applying it, and then updating the response to new information. And they're very rapid updaters. There is a saying that's very relevant to this whole thing which is that "life only makes sense looking backward, but it has to be lived going forward." My life has just been a quirky path dependent meander. I wound up doing this because I was recruited in a fluky way to a National Research Council Committee on American Soviet Relations in 1983 and 1984. The Cold War was at its height. I was, by far, the most junior member of the committee. It was fluky that I became engaged in this activity, but I was prepared for it in some ways. I'd had a long standing interest in marrying political science and psychology. Psychology is not just a natural biological science. It's a social science and a great deal of psychology is shaped by social context. [EDITORS NOTE: To volunteer for Tetlock's project in the the IARPA forecasting tournament, please visit www.goodjudgmentproject.com ] |
A SHORT COURSE IN SUPERFORECASTING
A SHORT COURSE IN SUPERFORECASTING
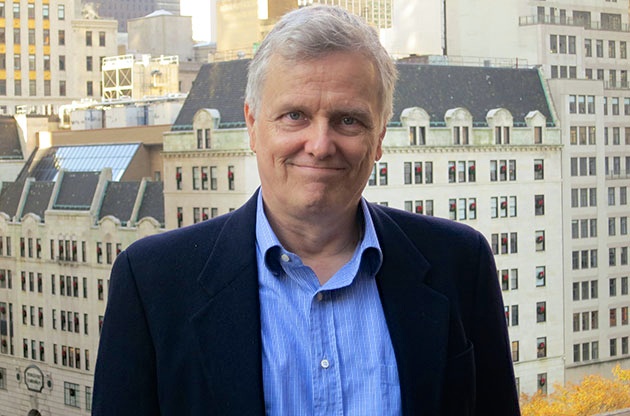
Edge Master Class 2015 with Philip Tetlock
— A Short Course in Superforecasting —
| Class 1 | Class 2 | Class 3 | Class 4 | Class 5 |
PHILIP E. TETLOCK is the Annenberg University Professor at the University of Pennsylvania, with appointments in Wharton, psychology and political science. He is co-leader of the Good Judgment Project, a multi-year forecasting study, the author of Expert Political Judgment and (with Aaron Belkin) Counterfactual Thought Experiments in World Politics, and co-author (with Dan Gardner) of Superforecasting: The Art & Science of Prediction.